The dress you are wearing is very nice(dress is beautiful).
→ The dress suits you very well (you are beautiful).
Share this transformation with more people.
Create an environment you can easily do clothes shopping anytime, anywhere and no matter whom you are with. And you can receive the object as like as two peas seen in the image.
Just stop “the picture is only for reference”, we don’t need it any longer.
People all over the world have experienced the same long stay at home because of covid-19. Today’s remote work and 5G environment have achieved so far, and the correspondence of the net sales becomes important in the apparel industry. In the past, you can attract customers attention simply by uploading goods catalog on the Internet. However, nowadays, consumers ask more. Differentiated experience is required to catch their attention and satisfy them. In this digital era, Personal oriented services such as virtual trying on is the best solution.
The purpose of trying on is to satisfy the shopping, so take improving the efficiency of trying on as a service.
(1) Confirmation of size and fit + (2) a good interpretation of you
→ (1) a good interpretation of you ・ confirm the suitable clothes (make a good match just in one step)
【provide high precision and reliable size and fit clothes】
Instead of just focusing on sales, turn your business to a more sustainable and appreciated mode. A mode in which consumers can design their own clothes and place the order after checking the VR fitting, then the production begins only when receiving an order. Everyone in this business cycle makes contribution to environment protection at the same time.
Sustainability and ethical shopping are familiar words to people now.
People begin to follow the principle in daily life and of course they keep sustainability in mind when they choose clothes.
Deeply loved clothes will be worn more often. Have you ever been hesitated when you want to discard your favorite clothes after wearing it? No matter how expensive the price is, the more times you wear it, in fact, the cost of each time you wear it decreases. The result is also very eco. This awareness is advanced.
Improving this awareness and changing people’s habit is not easy. Let’s start with tiny steps.
As for the mainstream 2D → 3D prototype (virtual garment production) in the apparel industry, I hope we can stop once and think about it.
Why do people have to take fitting in fashion stores? Why is the return rate of online selling clothes so high?
The answer is often referred to “fit & size” and “plus size”.
If there is no way to solve “fit & size” and “plus size” simultaneously, the high-precision “virtual trying on” service cannot be provided, and the result will inevitably be unable to connect to CX (customer experience).
What I want to draw attention to and emphasize here is that the method can solve “fit & size” and “plus size” simultaneously has already existed. (at least on the upper body)
As a human body, if there is no trunk, arms or legs can’t be existing independently. The essence of the “fit & size” problem is “generation of body trunk garment“, which must be created for covering the body trunk. About 8years ago, our Japanese company has obtained a method to solve the “fit & size” problem, named this as Clone size Ensured Technology (method). Since last year, SdibiT as our joint venture company in China has been kept striving to prove that this method is also applicable to “plus size”.
The decisive difference from the 2D pattern making up to now is the first stage of pattern making for generation of body trunk garment. No matter for which size. [2D pattern making method]and [2D grading method] are not used at all. The prototype of SdibiT is [3D (generate) → 2D → 3D (confirm)], named [Clone Shape Ensured Method] by us because of its extremely high 3D reproducibility of real clothes. That is to say, the comfort and shape of wearing are optimized at the same time, and the 3D virtual garment of the main body has been made in advance. Only by generating 3D virtual garment in advance, can we respond to evolving personalized customization requirement of the market. And it is also an indispensable engineering step to fulfill the personalized customization requirement. It has even been proved that the fine-tuning of higher and more accurate dimensions can be easily achieved by completing this step.
The foundation of the way of thinking is flattening 3D curved surfaces to 2D.
Reference: https://wiki.mcneel.com/labels/advancedflatting
Watch the animated video of the forepeak tank section of the hull. On the surface of 3D state presentation, where segmentation is needed can be known according to the places where extreme stretching or compression may occur. The degree of compression or stretch is determined by and reflected in the production of high-precision templates. We think this principle is also applicable to the production of 3D virtual garment.
Remember to play “paper craft of regional globe / wallpaper globe” when you were a kid, or football or rugby. To cover the sphere with a lot of small pieces of paper, it is difficult to paste and combine beautifully and accurately in practice. In fact, this is the same as the essence of garment pattern making.
Note 1): the paper globe is equivalent to a 3D virtual garment, not to an avatar or a digital torso(=virtual sewing body).
The garment can be considered as a surface full of curves. The line that divides the garment is the 3Dconstruction line of clothing. By flattening the segmented 3D slices, we can get the best approximate 2D slice plane pattern, which is the entrance to solve the “fit & size” problem. In order to make a difference from the 2D pattern commonly defined so far, we call the small piece of planar pattern (2D) obtained by flattening “2D flatten pattern”.
Note 2): on the 3D virtual garment, it is not just to set the 3D construction line of clothes freely and then generatethe2D flatten pattern. In order to control the tension or compression wrinkling in a certain range, it is necessary to correct the position of the3Dconstruction line of the clothes. The correction of this position will have a great influence on the 3Dreforming of clothes, including the sewing process.
Next, the specific examples of fashion design are illustrated.
To make the required 3D virtual garment on an avatar or torso, appropriate loosen setting should be given according to the statistical data or empirical data. The typical examples include air gap thickness.
Appropriate amount of looseness we meant here, can also be some stages or combinations, such as: tight / standard fit / easy fit.
No need to explain that loose quantity is different between men and women’s costume. The difference is also obvious between women’s cheongsam(chi-pao)and shirt blouse.
For example, Chinese cheongsam(chi-pao)is even considered as the second skin layer of women, with little looseness. In order to emphasize the beauty of women’s curve, some ladies pay attention to corrective undergarment or corset to correct their body shape, maintaining the significant S-shaped curve when they stand.
Here we explain simply the influence of different standing posture. When the horizontal cross-section shape of Bust / waist / hip overlaps (cross-section overlaps), you can see the change of the position relationship with Z coordinate. Then the outer circumference of the bust / waist / hip will also change. Even the height of Bust / waist / hip (Y coordinate) varies. That is to say, the posture of standing is different, the shape of avatar is also different. Therefore, it is necessary to think about the posture when wearing the clothes according to different clothing items and give appropriate loosen settings respectively.
It is possible for the instructors (professors, etc.) of college and other educational institutions or brand majors to make a sample garment according to different clothing categories by draping cutting. But it is difficult to keep the same shape if different 3D construction lines are used. In addition, the problem of grading (size expansion) cannot be solved efficiently and accurately in the traditional way, which also troubles many professionals. It is a too much donkeywork to figure out the method, however, that is just the essence of “fit & size” problem.
[example 1] the difference between 2D flatten pattern and 2D pattern in case of two darts being used.
Take women’s shirts which two darts being used for example to demonstrate.
Suppose you have created the required 3D virtual garment:
a) For the 3D virtual garment, follow the steps of 2D pattern making method to set the construction cutting line. First, add a dart from the front armpit to the chest point, then refer to the direction of the usual torso Princess line, and then add another dart at the waist (the second one). Thus, we can see that there are obvious tight stretching or compression parts on 2D flat pattern. (Strong bias area / deformation ratio)
b) For the 3D virtual garment, as in a), first add a dart from the front armpit to the chest point. Meanwhile, a 2D flatten pattern is generated, so you can see the place where there is excessive tension, stretching or compression wrinkling. In order to eliminate this kind of excessive scaling, the second dart is added and regenerated into a 2D flatten pattern. Compared with a), it can be found that the small flatten pattern obtained at this time has greatly reduced the expansion strength.
Conclusion: the2D flatten pattern obtained by using the method of B) can be quite close to the 3D virtual garment required. And from the pointof the feasibilityof production and
processing, it is also close to the best data.
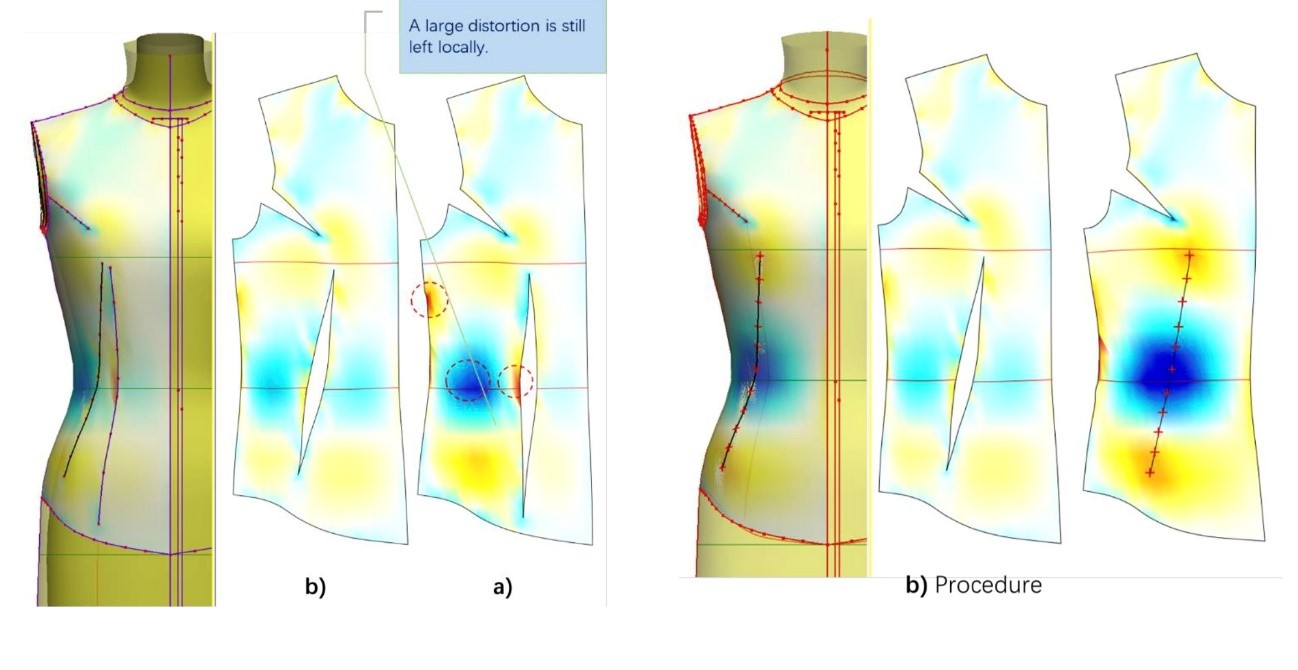
However, just like this, the “fit & size” problem has not been completely solved. As a part of the clothing construction line b), how to decide the position of the second dart, whether it make the part look beautiful (whether it is against the sense of appearance), is still left behind. From the perspective of production and manufacturing, the expansion ofthe2D flatten pattern will not cause problems, and the position of darts should be corrected to a position that can take into account the aesthetic. This is the limit of manufacturing requirement.
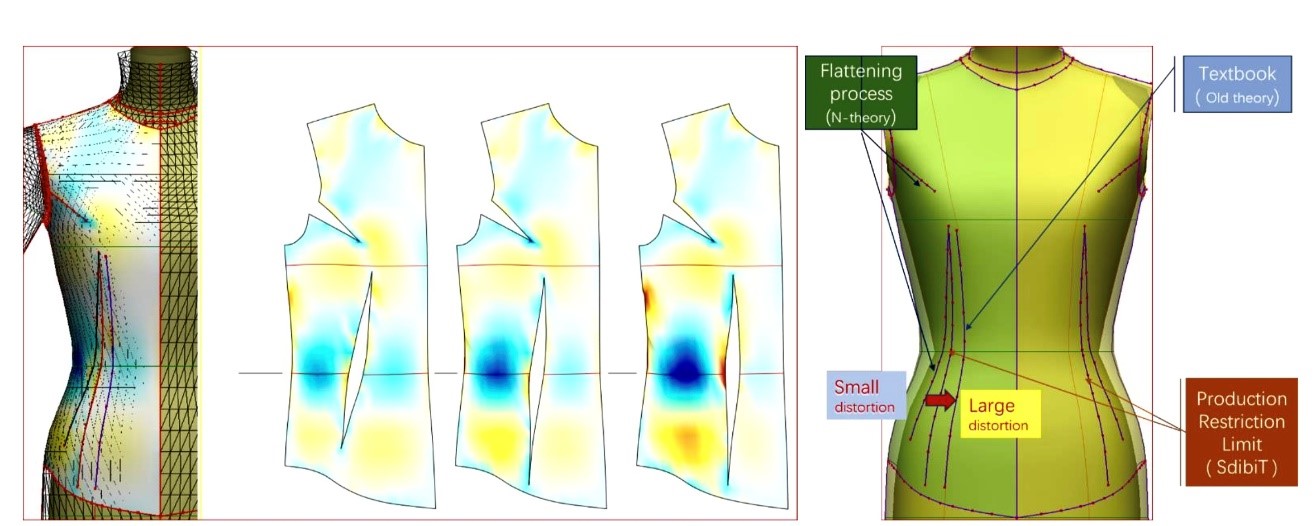
Obtaining the 2D flatten pattern that can match the manufacturing constraints is the way to completely solve the “fit & size” problem.
[example 2] corresponding to personalized customization
13T and 12TL are the ready-to-wear (RTW) sizes of SdibiT and are actually on sale. Here we use the torso of women’s shirt and the bust cross section of 3D virtual garment to demonstrate the description. Here is the actual body shape data of a person (HH) and a 3D virtual garment chest cross section based on personalized customization.
In daily life, HH is accustomed to wear a bra (push up bra) with lifting effect. Both 13T and 12tl in RTW size can be worn by her in fact. However, HH is not particularly satisfied with neither of the two sizes by trying on comparing.12TL for her, there is not enough space in front and back, and the cup shape of her bra appeared slightly. But 13T for her, loosing on both sides of her left and right armpits, hardly reflects the optimization effect on her body shape brought by the lifting bra. That is to say, the most suitable shirt for her is the shirt (3D shape) with a width of 12TLfrom left to right and a thickness of 13T from front to back, which can make the optimization effect of the bra outstanding. In addition, HH also has her own preference and stress on the cutting line of fabric switching part.
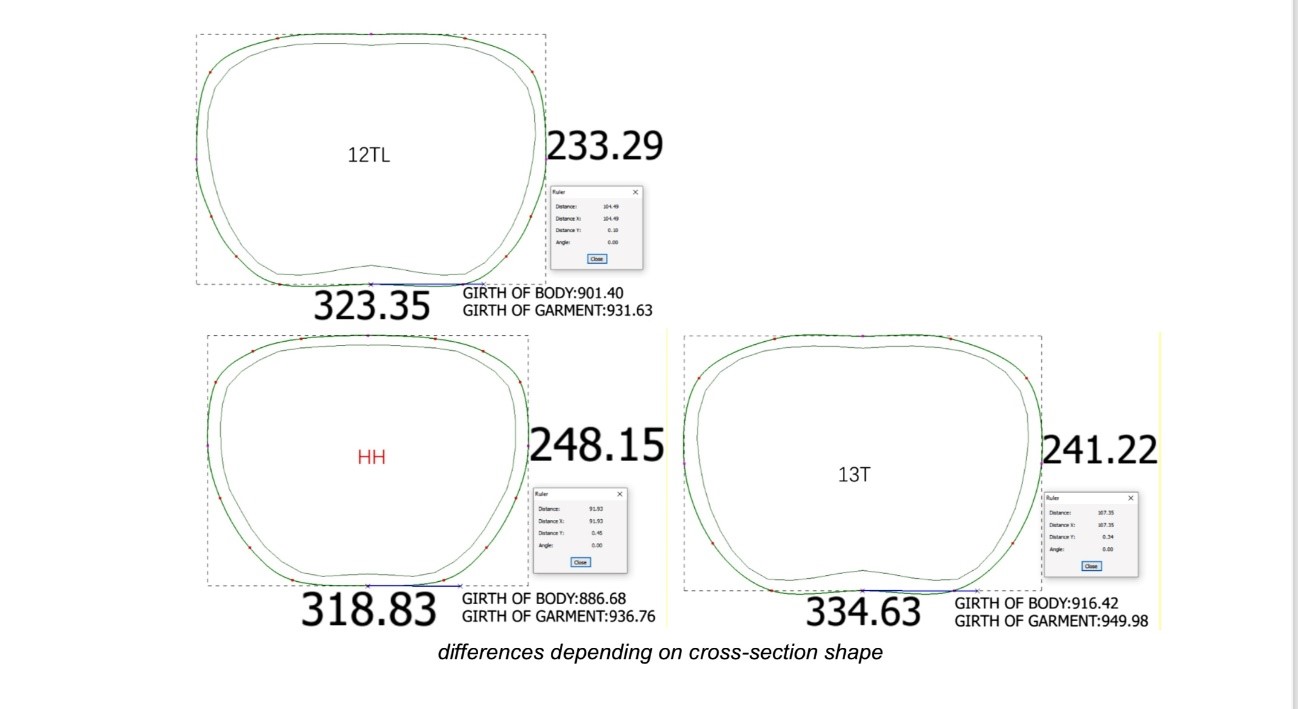
According to the 3D shape expected by HH, 3D virtual garment is made, and the 3D construction lines that she likes are adopted. Compared with 12TL and 13T, the best position of the garment 3Dconstruction line in the processing restriction range is about 10 mm closer to the front center of the chest cross-section line.
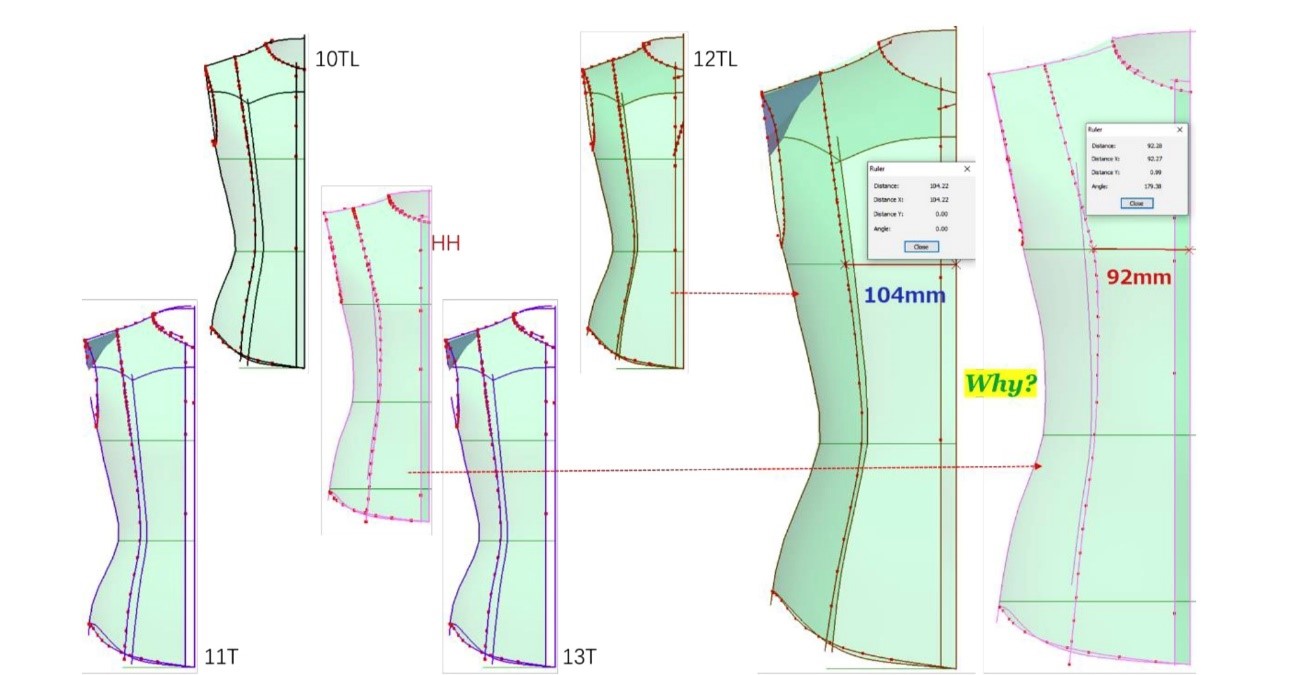
Referring to the position of 12TLor 13T garment 3Dconstruction line viewed from the front direction, when it is adjusted to the position with beautiful appearance, the combination of three small pieces including the front fly is in the restriction range of subsequent processing. However, there are only two pieces of pattern combination, without the single part of front fly, there will be high possibility of problems in sewing. If the 3Dconstruction line of clothing is within the limit of processing restriction, even without the single part of front fly, there is no problem with only two pieces pattern combination.
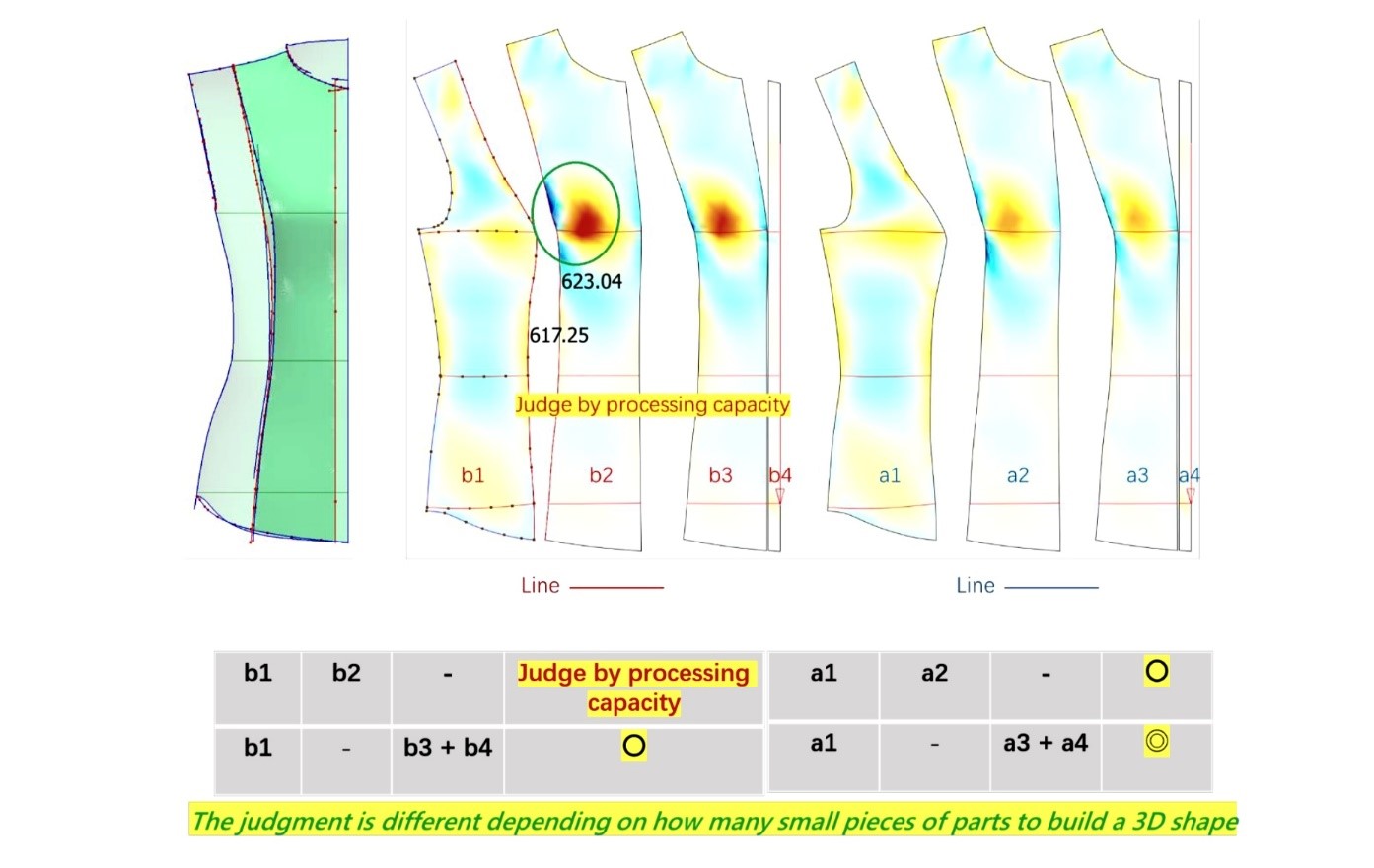
That is to say, for the same 3D garment, the shape and structure of the forepart of the upper body are processed according to the combination of 2 pieces (2 parts) or 3 pieces (3 parts), the position of the 3Dconstruction line of clothing in the production restriction range will be different.
To solve the “fit & size” problem, before 2D → 3D prototype (virtual clothing production), it is necessary to determine the position of 3D construction line of clothing that can take into account both the aesthetic and manufacturing constraints on the basis of 3D virtual garment, and then generate 2D flatten pattern.
————————————————————————————————————————
In the lectures for instructors (professors, etc.) of collegesand other education professionals, it will be emphasized that:
1.Shape determines position
・The 3D virtual garment determines the position of the 3Dconstruction line of clothing within the production restriction limit.
・The 3D construction lines of clothes are mutually restricted and influenced
2.The position determines the amount of suture ingestion(for ease stitch sewing)
・Change the 3Dconstruction line of the clothes, and the amount of contradiction sewing(contraction quantity)required will also change.
Furthermore, in the lectures for employees of clothing enterprises, emphasis follows will be put on:
3.The enterprise should determine the benchmark to maximize the general design
・3D virtual garment should be set as the benchmark, as well as the 3Dconstructionline of clothing that can take into account both aesthetic and limit of production restriction should be determined by the organization that leading the brand operation.